Don’t dismiss GPT-3/Stable Diffusion use cases if they don’t work right away
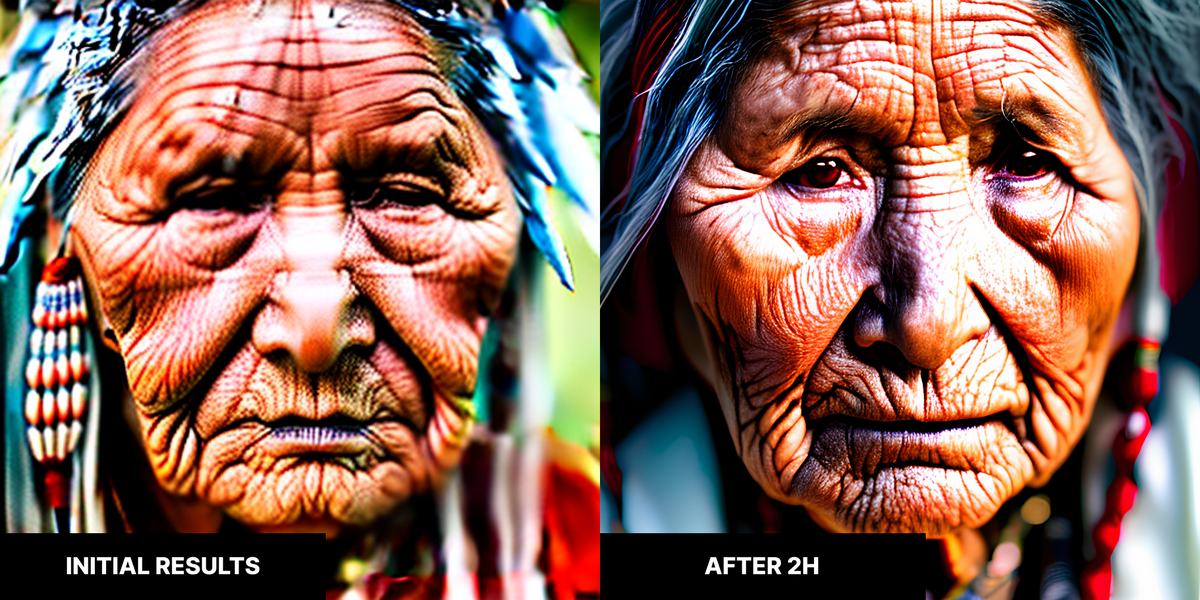
Over the past two years, I’ve seen the following happen at least a hundred times:
A person has an idea how to use one of the foundation models. Say, Stable Diffusion to generate 2D avatars for a mobile game. They open one of the tools, try creating an image they need, fail to achieve human-level quality in ten minutes, and quit in despair, tweeting that “your models are just toys and never gonna be used in production!!”
Hell, I’ve committed this fallacy too. The most dramatic case was when I dismissed the idea of an AI copywriter SAAS two years ago because I couldn’t make vanilla GPT-3 generate texts comparable to those of a human writer in a few days of work. We all know how that played out: Jasper has just raised $125m Series A and is one of the fastest-growing startups ever, and Copy.ai has just crossed $10.8m ARR.
Why do people give up so easily? Why did I give up?
One reason is unreasonable expectations. Because of all the hype around GPT-3 and now DALL-E and Stable Diffusion, because of all the cherry-picked demos on Twitter, myself and others were and still are coming to this tech with hyper-inflated expectations. We think it can do anything, EVERYTHING, right away. Naturally, we get terribly disappointed when it doesn’t work that way.
Another reason is the deceptive simplicity of the interface. In most cases, people first try foundation models through a text box where you type stuff, click a button, and magic happens (or so they say). In fact, as Pieter Levels noted here, real magic never happens that way — it takes days/weeks/months of prompt-engineering, fine-tuning on your own data, and stacking models together like Lego blocks. But this simplistic UI tricks us into believing otherwise, and we pay for it.
So, how does one get around this fallacy?
Simply being aware of these two reasons helps; I can testify to that. For instance, when I tried generating b&w portraits of elderly native American women with Stable Diffusion 2 a few days ago, the first results were crap. After 2h of tinkering, however, I’ve accomplished much better quality.
What’s even more helpful, however, is a change of tactic one employs to figure out what to do with GPT-3, Stable Diffusion, DALL-E, and other models. Instead of thinking, “Let’s try these models and see in which use cases I can get acceptable results quickly” one may think, “Let’s pick a seemingly lucrative use case that I’m interested in and try to figure it out, even if that takes some time.” In other words, to focus on the benefits one may get rather than on the cost in time to create a thing. A good example is, again, Pieter Levels and his AvatarAI project. It took him good 2-3 weeks to make Stable Diffusion generate top-notch avatars, but the outcome was obviously worth it: the project made $100k in the first week after launch and is still making $11k per week even though the initial interest faded out.
I admit: this is incredibly hard to do in the FOMO Twitter world, where everybody and their brother seem to be starting AI-first unicorns every day. But history teaches us that FOMO-inspired actions rarely lead anywhere and that calm and steady always wins the race. I bet it’s no different here — we just have to keep this idea in mind at all times and act on it.